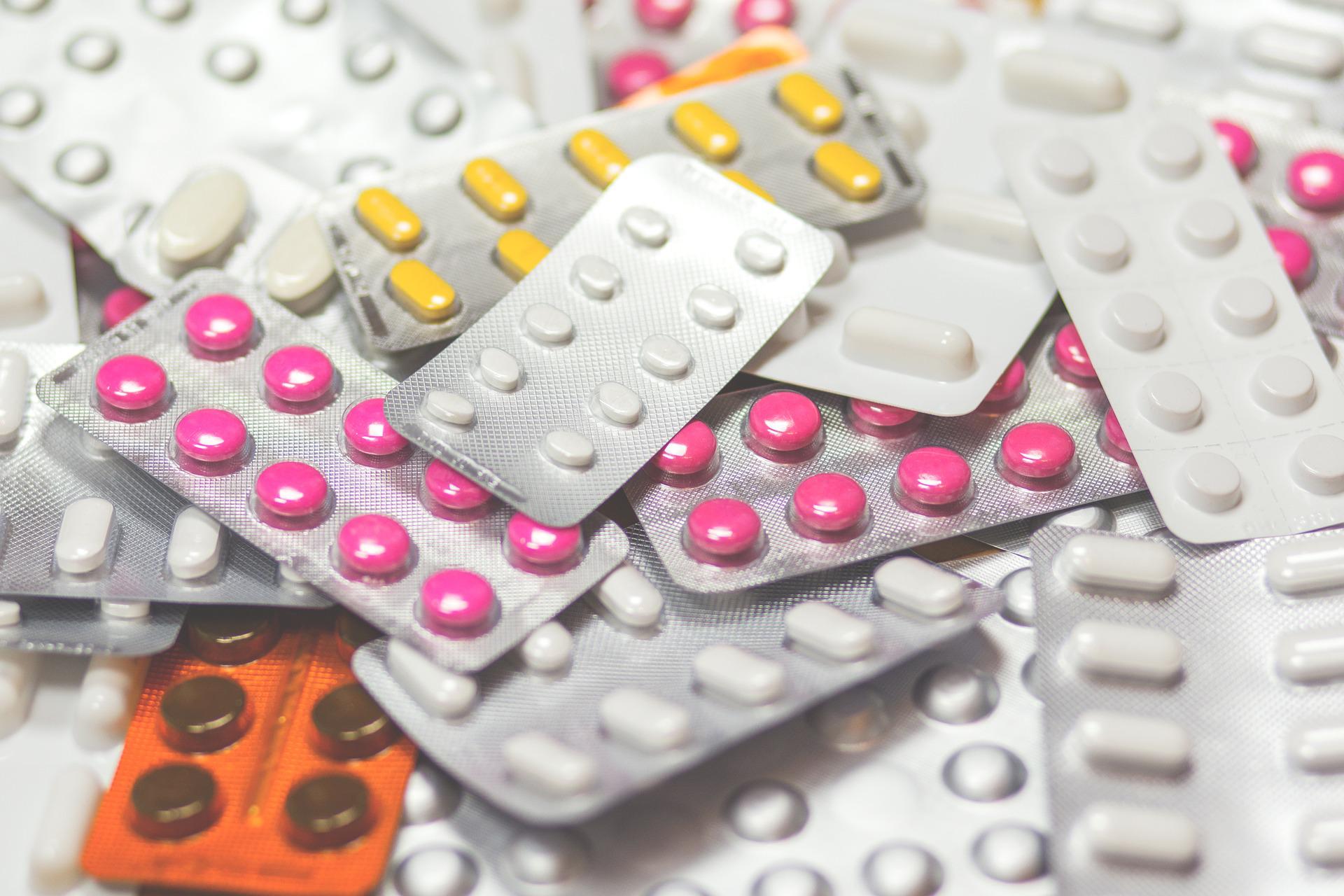
High-throughput prediction of human organ toxicity
Nephrotoxicity Prediction
This innovative method (Su et al. 2016) was awarded the Lush Science Price 2016 and combines cell-based assays with bioinformatics. The method predicts specifically toxicity to renal proximal tubular cells, which are one of the main targets for chemical-induced toxicity in the kidney. The method can be used with human primary renal proximal tubular cells (PTC), human kidney-2 (HK-2) cells (established proximal tubular cell line), or induced pluripotent stem cell (iPSC)-derived PTC-like cells (Kandasamy et al. 2015) cultivated in 384-well plates. Cells are imaged by high-content imaging after compound exposure. Chemical-induced toxicity to PTC in humans is predicted based on chemical-induced changes of predictive cellular phenotypic features, which were identified by phenotypic profiling and machine learning. With human primary PTC, the method has a sensitivity of 87% and a specificity of 90%. Machine learning and 10-fold cross-validation were applied for the predictive performance analysis. The method was applied in a case study on triazole fungicides initiated by the National Institute for Public Health and the Environment (The Netherlands) (van der Ven et al. 2020) and for the prediction of the nephrotoxicity >300 ToxCast chemicals in collaboration with the US Environmental Protection Agency.
Prediction of hepatocyte toxicity in humans
This high-throughput method (Hussain et al. 2020) works with HepaRG™ cells in 384-well plates. Similar to the method for nephrotoxicity prediction it is based on a combination of high-content imaging, phenotypic profiling, and machine learning. The method has been validated with 69 reference chemicals that were known to be toxic or not toxic for hepatocytes in humans. It has a test sensitivity of 73% and a test specificity of 92%. Machine learning and 10-fold cross-validation were applied for the predictive performance analysis.
Prediction of vascular endothelial toxicity in humans
This method based on high-content imaging has been validated with a reference set of 32 chemicals that are known to be toxic or not toxic for human vascular endothelial cells. It has a sensitivity and specificity of 89% and 96%, respectively. The method was established with human umbilical vein endothelial cells (HUVEC), and similar results were obtained with 3 other types of primary human vascular endothelial cells.
Key highlights
High accuracy
High throughput
Toxicity prediction by machine learning
Not restricted to specific classes of chemicals
Award-winning
Applied in collaborations with regulators
How is the human organ toxicity of your test compounds predicted?
You send your test compounds.
We apply your test compounds in the high-content screening assay(s) of your choice. Typically, 7 (minimum 5) concentrations of each chemical are screened with 3 technical replicates per condition and experiment. Positive and negative plate controls and vehicle controls are included. Compound solubility at the tested concentrations will be assessed.
The prediction of human organ toxicity of your test compounds is based on the bioinformatics analysis of the high-content imaging data.
You receive the toxicity prediction for all tested compounds and a report on all results.
References
Hussain F, Basu S, Heng JJH, Loo LH, Zink D (2020) Predicting direct hepatocyte toxicity in humans by combining high-throughput imaging of HepaRG cells and machine learning-based phenotypic profiling. Arch Toxicol 94(8):2749-2767 doi:10.1007/s00204-020-02778-3
Kandasamy K, Chuah JK, Su R, et al. (2015) Prediction of drug-induced nephrotoxicity and injury mechanisms with human induced pluripotent stem cell-derived cells and machine learning methods. Sci Rep 5:12337 doi:10.1038/srep12337
Su R, Xiong S, Zink D, Loo LH (2016) High-throughput imaging-based nephrotoxicity prediction for xenobiotics with diverse chemical structures. Arch Toxicol 90(11):2793-2808 doi:10.1007/s00204-015-1638-y
van der Ven LTM, Rorije E, Sprong RC, et al. (2020) A Case Study with Triazole Fungicides to Explore Practical Application of Next-Generation Hazard Assessment Methods for Human Health. Chem Res Toxicol 33(3): 834-848 doi:10.1021/acs.chemrestox.9b00484